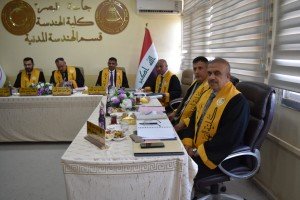
A master's thesis at the College of Engineering at the University of Basrah discussed energy demand modeling based on deep learning to predict fluctuations in the demand response application.
The thesis submitted by student Sarab Shannan Sawadi explained how the integrated machine learning process can significantly increase forecasting accuracy. It showed how well XGBoost and Random Forest performed individually and as a group.
It intends to build a hybrid deep learning strategy (RNN-GRU), which is based on sequential learning and uses both recurrent neural network (RNN) and gated recurrent units (GRU). Several deep learning models have been used, including single-layer RNN, single-layer GRU, stacked RNN, stacked GRU.
The thesis concluded that evaluating the experiment results using mean absolute percentage errors (MAPE) and accuracy (R2-Score) methodologies revealed that the predictions of the newly constructed model are the most efficient with the lowest error rates, with MAPE = 5.6945 and R2 = 94.3055, respectively.